We all know that in today’s world, everyone is talking about AI, its impacts, and how to use AI for various purposes. If you are excited to know the best budget GPU for AI or if you are looking to know how to use AI for budgeting, then you are on the right page.
In this detailed article, we will explore the resources and how to use those resources to succeed in today’s world. Stay tuned.
Introduction: best budget GPU for AI
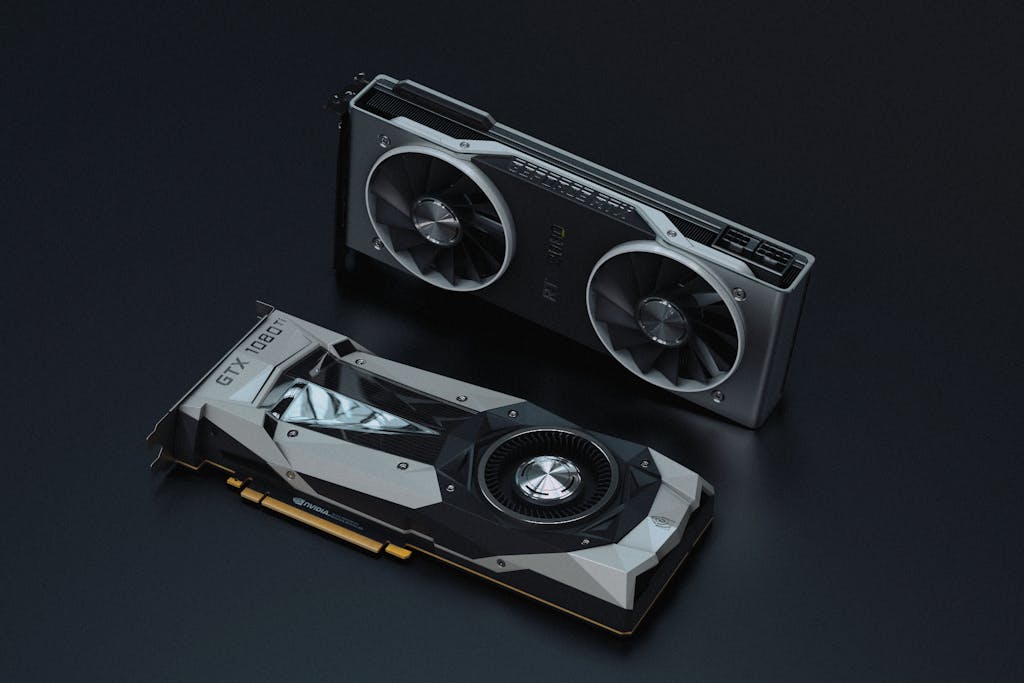
When it comes to finding the best budget GPU for AI applications, it’s essential to consider performance, cost-effectiveness, and compatibility with AI tools. A reliable GPU can significantly enhance the speed and efficiency of AI algorithms, improving financial forecasting, data analysis, and decision-making processes. By investing in the right GPU for AI, individuals and organizations can take advantage of advanced features and capabilities to streamline budget management tasks. From optimizing budget allocations to enhancing risk management strategies, the right GPU can make a significant impact on the effectiveness of AI-powered budgeting tools. In this article, we will explore the top considerations for choosing the best budget GPU for AI in budgeting applications.
The Evolution of GPUs in AI Applications
In the realm of budgetingand financial management, the use of GPUs in AI applications has seen significant evolution. When it comes to finding the best budget GPU for AI tasks, cost-effective options that offer powerful computational capabilities are essential. These GPUs play a crucial role in accelerating the processing of complex algorithms and datasets, ultimately enhancing the efficiency of AI tools for budgeting.
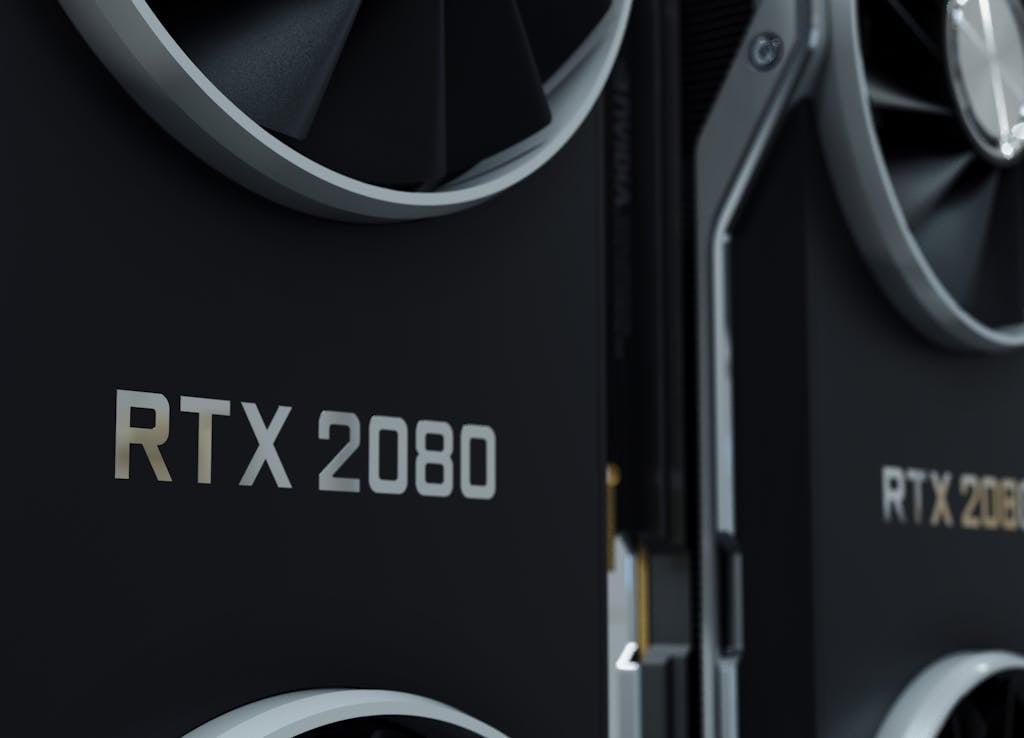
Whether it’s for personal finance management or organizational budget management, having the right GPU can significantly impact the speed and accuracy of AI-driven tasks. As AI continues to advance in the financial sector, the demand for budget-friendly GPUs with robust performance for budgeting applications is on the rise.
Understanding GPU Specifications for AI
When it comes to selecting the best budget GPU for AI applications, it’s important to consider several key specifications that can impact performance and efficiency. Understanding these specifications can help you make an informed decision when choosing a GPU for your AI projects.
Core Count and Processing Power
One of the most important specifications to look at when choosing a GPU for AI is the core count and processing power. The core count refers to the number of cores within the GPU that are responsible for processing data. In general, the higher the core count, the more processing power the GPU has, which can result in faster AI computations. When looking for a budget GPU for AI, it’s important to find a balance between core count and cost to ensure you’re getting the best performance for your budget.
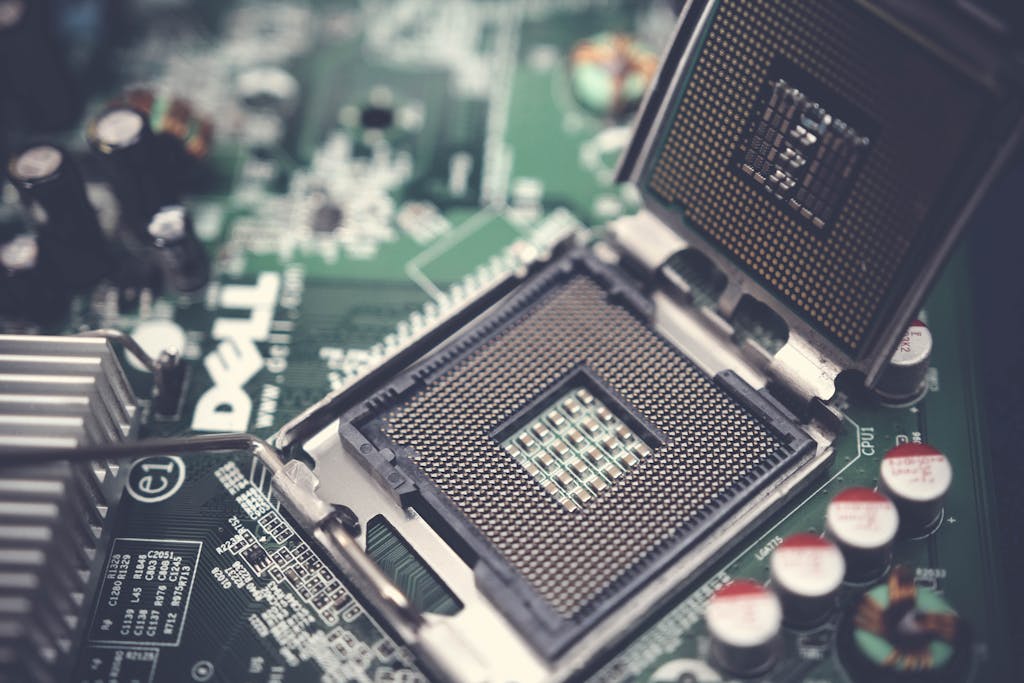
The best budget GPU for AI
Memory Size and Bandwidth
Memory size and bandwidth are also crucial specifications to consider when selecting a GPU for AI applications. The memory size determines how much data the GPU can store and access quickly, while the memory bandwidth determines how quickly data can be transferred to and from the GPU. For AI workloads that involve processing large datasets, a GPU with ample memory size and high memory bandwidth is essential to ensure smooth performance. When looking for a budget GPU for AI, consider your specific AI tasks and data requirements to determine the optimal memory size and bandwidth for your needs.
Power Efficiency and Cooling Requirements
Power efficiency and cooling requirements are often overlooked but important specifications to consider when choosing a budget GPU for AI. GPUs can consume a significant amount of power, so selecting a power-efficient GPU can help reduce energy costs and minimize heat generation. Additionally, AI workloads can be intensive and generate a lot of heat, so proper cooling is essential to prevent thermal throttling and ensure optimal performance. When choosing a budget GPU for AI, look for models that offer a good balance between power efficiency and cooling capabilities to maximize performance while staying within your budget constraints.
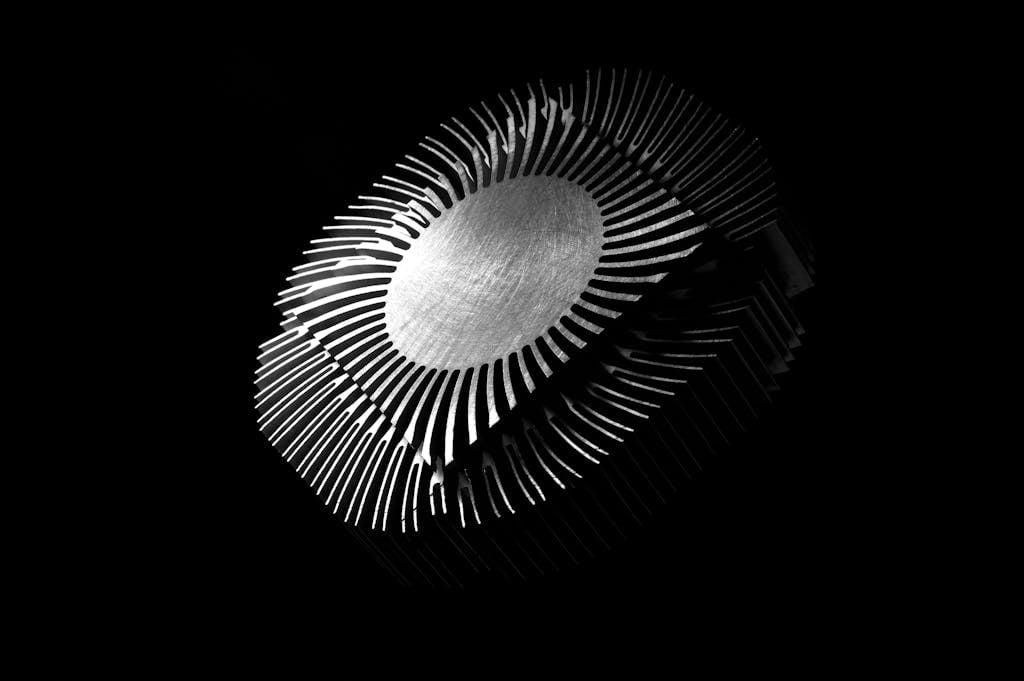
By understanding these key specifications for GPUs in AI applications, you can make informed decisions when selecting the best budget GPU for your specific AI projects. Consider your core count and processing power needs, memory size, and bandwidth requirements, as well as power efficiency and cooling capabilities, to ensure you’re getting the best performance for your budget.
Top Budget GPUs for AI Projects in 2024
When it comes to AI projects, having the right GPU can make a significant difference in performance and efficiency. In 2024, several budget-friendly options will offer a good balance of performance and affordability. Here are some top picks for budget GPUs for AI projects:
Entry-Level Options
Here we collect some of the best options available to choose and we hope you would like to check out.
- NVIDIA GeForce GTX 1650: This entry-level GPU from NVIDIA is a great option for budget AI projects. It offers decent performance for tasks like image processing and machine learning, making it a solid choice for beginners.
- AMD Radeon RX 5500 XT: AMD’s Radeon RX 5500 XT is another affordable GPU that is well-suited for AI projects on a budget. With good computing power and memory bandwidth, it can handle basic AI workloads effectively.
- NVIDIA Quadro P1000: While technically a workstation GPU, the NVIDIA Quadro P1000 is a cost-effective option for those looking to dip their toes into AI projects. It offers reliable performance and stability for tasks that require precision and accuracy.
Mid-Range Contenders
- NVIDIA GeForce RTX 2060: Moving up to the mid-range segment, the NVIDIA GeForce RTX 2060 is a solid choice for AI projects that demand more processing power. With dedicated AI cores and ray-tracing capabilities, it can handle more complex AI workloads effectively.
- AMD Radeon RX 5700 XT: AMD’s Radeon RX 5700 XT is another strong contender in the mid-range GPU market. With a high core count and fast memory, it excels at AI tasks that require parallel processing and high bandwidth.
- NVIDIA Quadro RTX 4000: For those looking for professional-grade performance without breaking the bank, the NVIDIA Quadro RTX 4000 is a worthy option. It offers a good balance of computing power and memory capacity for mid-range AI projects.
When choosing a budget GPU for AI projects in 2024, consider factors like compute power, memory bandwidth, and specialized AI features. While these entry-level and mid-range GPUs may not offer the same performance as high-end models, they provide a cost-effective solution for beginners and those working on small to medium-sized AI projects.
Factors to Consider When Choosing a GPU for AI
When selecting a GPU for AI applications, there are several key factors to consider to ensure optimal performance and efficiency. One of the most important considerations is the GPU’s computing power, which determines how quickly it can process complex AI algorithms and models. Look for GPUs with high CUDA core counts and memory bandwidth to handle AI workloads effectively.
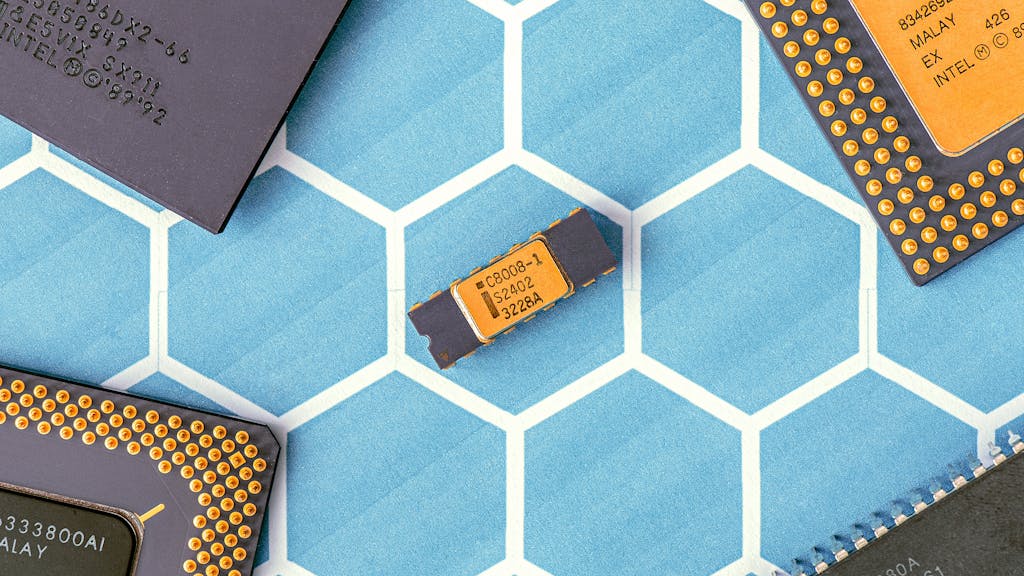
Another crucial factor to consider is the GPU’s memory capacity. AI models often require large amounts of memory to store and process data efficiently. Choose a GPU with ample VRAM to prevent bottlenecks and ensure smooth operation when working with large datasets or complex neural networks.
Additionally, consider the GPU’s compatibility with popular AI software and tools. Some GPUs may offer optimizations or support for specific frameworks like TensorFlow or PyTorch, allowing for better performance and compatibility with your existing AI workflow.
Compatibility with AI Software and Tools
Ensuring that your chosen GPU is compatible with the AI software and tools you plan to use is essential for seamless integration and optimal performance. Check for drivers and SDK support from the GPU manufacturer for popular AI frameworks and libraries. This will help avoid compatibility issues and ensure that you can leverage the full capabilities of your GPU for AI tasks.
Furthermore, consider the flexibility and scalability of the GPU when it comes to supporting future updates and advancements in AI technology. Look for GPUs that are regularly updated with new features and optimizations to stay ahead of emerging trends in AI development.
Future-Proofing Your Investment
Investing in a GPU for AI should not only meet your current needs but also provide room for growth and adaptation as your AI projects evolve. Consider future-proofing your investment by selecting a GPU with a balance of performance, memory capacity, and compatibility with emerging AI technologies.
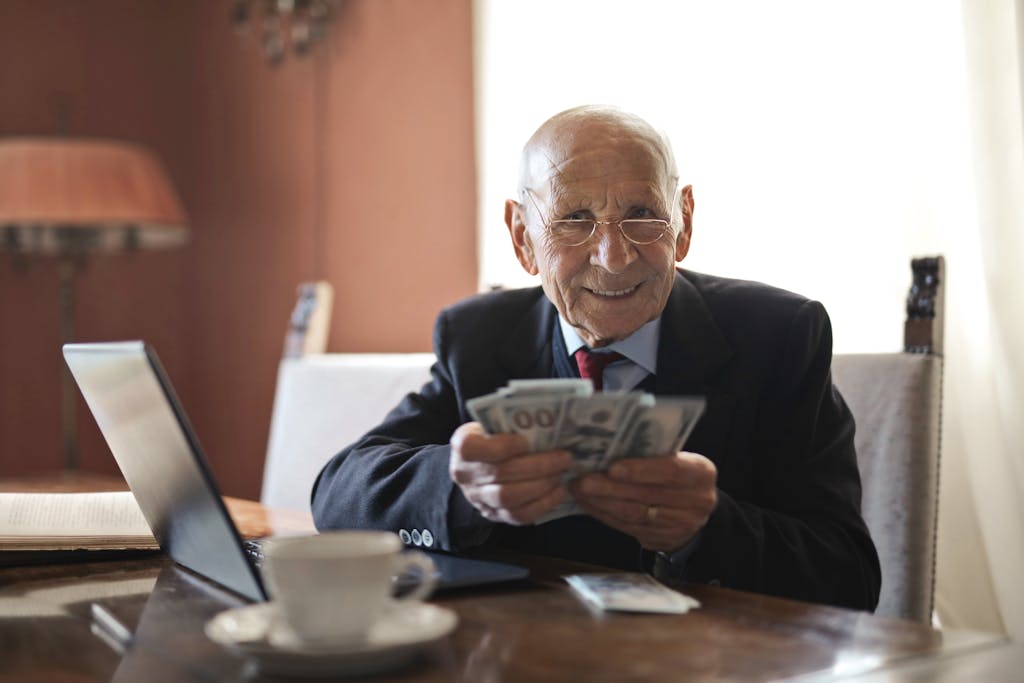
Future proof Investment
Look for GPUs with a track record of reliable performance and consistent updates to ensure longevity and support for future AI applications. Additionally, consider factors like power efficiency and cooling capabilities to ensure that your GPU can handle intensive AI workloads without overheating or consuming excessive energy.
By carefully considering these factors when choosing a GPU for AI applications, you can optimize performance and compatibility and future-proof your investment for long-term success in your AI projects and budgeting initiatives.
You may also read: How to Make Money with Chat GPT Reddit: A Complete Guide
Conclusion: Balancing Cost and Performance
When selecting the best budget GPU for AI applications, it is crucial to strike a balance between cost-effectiveness and performance. While there are numerous options available in the market, considering factors such as computational power, memory capacity, and compatibility with AI tools is essential. Look for GPUs that offer a good combination of affordability and efficiency to optimize your budgeting processes effectively.
By investing in a cost-effective GPU that meets your AI requirements, you can enhance your budget management tasks and streamline data analysis for better decision-making. Remember to research thoroughly and compare different options to find the most suitable GPU that aligns with your budget constraints and performance needs in the realm of AI for budgeting.
Frequently Asked Questions
What GPU to use for AI?
High-end NVIDIA GPUs like RTX 30 series or Quadro RTX are popular choices for AI due to their powerful computational capabilities and dedicated AI-oriented features.
Do I need a GPU for AI?
While not strictly necessary, GPUs significantly accelerate AI training and inference processes, reducing time and computational costs compared to CPU-only setups.
How much GPU for AI?
The amount of GPU required for AI depends on the complexity and scale of the tasks. For small-scale projects, a mid-range GPU may suffice, while larger projects may require high-end GPUs with more computational power.
What is the minimum GPU for AI training?
A minimum of 8GB VRAM is generally recommended for AI training tasks to accommodate larger datasets and complex neural network models efficiently.
Is Nvidia better for AI?
NVIDIA GPUs are widely regarded as better suited for AI due to their optimized architecture, extensive software support, and dedicated AI features like Tensor Cores.
Is AMD or Nvidia better for AI?
While AMD GPUs offer competitive performance, NVIDIA remains the preferred choice for AI due to its established ecosystem, robust software support, and specialized AI hardware.
Is a 2GB graphics card enough for AI?
A 2GB graphics card is likely insufficient for most AI tasks, as AI workloads often require larger memory capacities to handle complex computations and datasets effectively.
Why GPU is best for AI?
GPUs excel in parallel processing, making them ideal for AI tasks that involve vast amounts of data and complex calculations. Their high throughput and optimized architecture significantly accelerate AI training and inference processes compared to traditional CPUs.
I don’t think the title of your article matches the content lol. Just kidding, mainly because I had some doubts after reading the article.
I’m sorry to hear you had doubts after reading the article. Let me know what specifically confused you, and I’ll do my best to address it. Your feedback helps me improve!”
Thank you for your sharing. I am worried that I lack creative ideas. It is your article that makes me full of hope. Thank you. But, I have a question, can you help me?